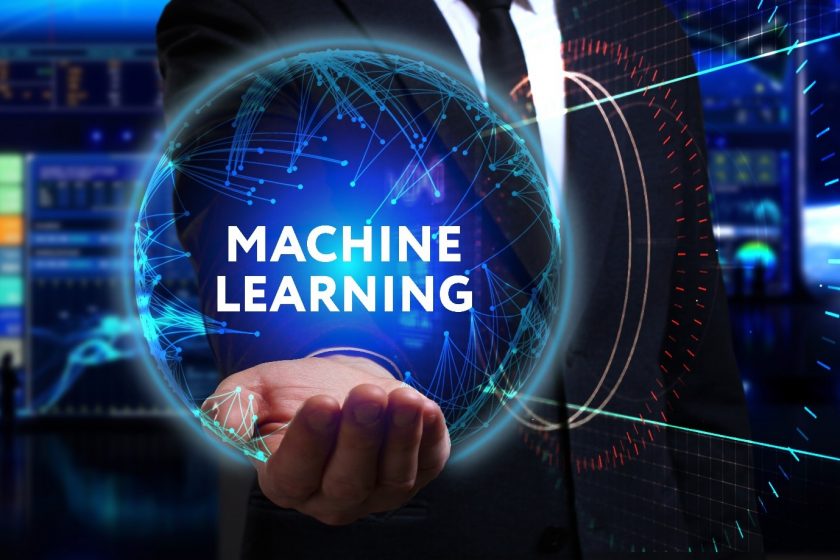
EVOLVING ROLE OF MACHINE LEARNING IN SPORTS GAMING
It is no secret that Artificial Intelligence (AI) is revolutionizing every industry on earth, but many people are not sure exactly how or why. For most, the term “AI” conjures an image of Arnold Schwarzzenager playing The Terminator, which may be the cause of such confusion.
The forms of AI that exist today are referred to as “Narrow AI” in that they are programmed with a specific objective to accomplish and become incredibly efficient at accomplishing only that objective. This stands in contrast to “General AI” or “Artificial General Intelligence” which describes the class of machines that can interact with the world just as humans do across all dimensions.
The best way to think about Artificial Intelligence in its modern applications would be in as an incredibly powerful and efficient tool for recognizing patterns and making predictions. Machine Learning (ML) is a subfield within the AI framework in which algorithms are able to iteratively optimize themselves, often without human oversight.
Across the sports gaming landscape, there are a number of key use cases in which Machine Learning is already being applied.
-
Oddsmaking
The heart of any sports betting operation is the odds at which various propositions are wagered on. With increasingly-large arrays of available data, the ability to leverage extremely efficient, highly predictive algorithms allows operators and suppliers to generate pre-match and in-play odds with more clarity and less latency. Not only does this boost profitability in the short-term, but also enhances the user experience and dramatically increases customer retention over the long-term.
-
Risk Management
Ultimately, operators are in the business of managing risk. This is often made difficult by outdated pricing models that rely on human input and are suboptimally configured given various operational constraints. With an ML framework, risk managers will not only be able to update predictive models in real time, but also anticipate behavioral patterns among active users in order to optimize and streamline functionality.
-
Responsible Gaming
Responsible Gaming, refers to the suite of mechanisms by which customers are kept from the ills of addictive behaviors. Almost unilaterally, this is limited to self-exclusion and self-imposed restriction tools. The term, “Responsible Gaming” may need to be replaced by “Sustainable Gaming,” because the latter more accurately and effectively communicates the notion that protecting customers from gambling addiction while preserving the integrity of underlying sporting events does not only benefit a few individuals, but every single stakeholder in the sports gaming industry, and even sports entertainment industry, more broadly.
Layering Machine Learning engines on top of robust behavioral profiles will grant unprecedented abilities to detect deviations from sustainable gaming behavior in real time and implement a solution before the problem even starts.
-
Bet Recommendations
Bet recommendations are similar to the functionality through which Netflix keeps you watching and Facebook keeps you scrolling. Right now, every customer that logs on to an online sportsbook is shown the same promotions. However, knowing any of the following, as examples, could help operators create more customized, curated, and likely profit-maximizing user flows:
- A particular customer enjoys having their winning payouts boosted as opposed to parts of their losses refunded
- The book is underexposed to a team that plays in a geography in which a large number of customers are currently online
- A customer cohort has an affinity for a particular bet type
-
Fraud Detection
Attempts at fraud are rampant in the industry for a number of reasons, and those attempting to perpetrate these frauds are growing more and more technically sophisticated. Accordingly, it is going to quickly become vital to leverage Machine Learning for detecting patterns that signify syndicate betting, bonus system exploitation, betting on behalf of 3rd parties, and arbitrage seeking. Generally speaking, KYC/AML implementations will advance exponentially with the combined application of high-fidelity data sets and high-speed algorithms.
Cause for Concern
The deployment of machine learning in this space will add massive value for a large number of stakeholders, but that does not mean it should be applied haphazardly.
One key area of concern is this idea of “backdoor functionality” whereby third-party technology companies that license out their software to sportsbook operators, typically in white label fashion, can sometimes embed the ability to scrape things like customer data without that operator being aware. The black box problem is not specific to sports betting and exists in virtually all fields where AI is being applied. It describes the issue of being unable to trace the source of unexpected results due to the incredibly complicated nature of the algorithms. Flash crashes caused by AI have already presented themselves in financial markets and could, theoretically manifest in sports betting markets as well. Finally, Odds Manipulation and Fraud Masking are merely symptoms of the fact that, just as much as AI and ML can be used by the “good guys”, it can be used adversarially by the “bad guys” as well.
Laying on top of, or perhaps parallel to those concerns, is the unfortunate fact that the high demand for AI products along with their complex nature has led to an environment in which many companies falsely advertise solutions that are far less sophisticated and effective than they purport to be.
Conclusion
In summary, it is unquestionably the case that data will be the most valuable resource on earth in the 21st century. Sports gaming and analytics are two of the most heavily data-laden industries in the entire world. As such, Lloyd. That said, the widespread acceptance of AI technologies brings with it a host of confusing ethical issues and business problems that will need to be approached tactfully and with maximal transparency and accountability.
Lloyd Danzig, Chairman & Founder ICED(AI)